Jun 27, 2024
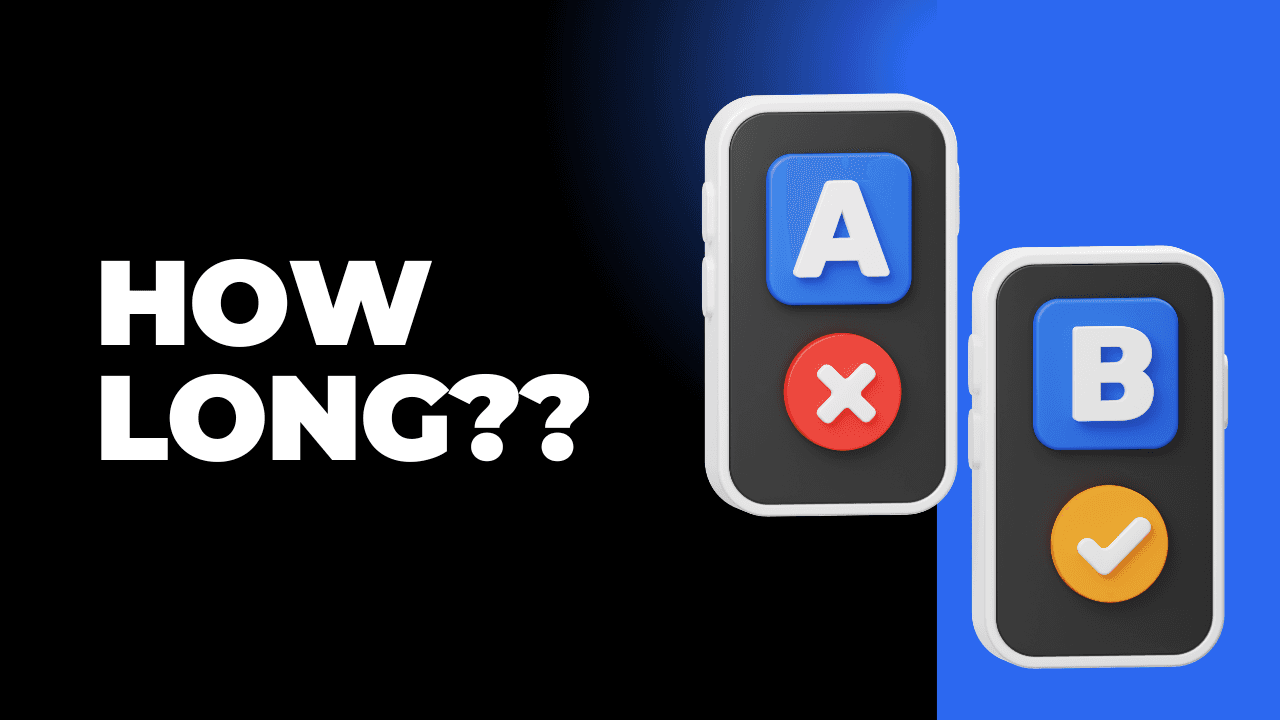
AB testing is an essential tool for optimizing webpages, emails, app features, and other elements by comparing two versions to see which performs better. One of the most common questions about AB testing is how long it should take to run. The duration of an AB test is critical for obtaining accurate, reliable results that can drive effective decision-making. This guide will explain the factors that influence the duration of an AB test and provide insights on how to determine the appropriate length for your tests.
What is AB Testing?
AB testing, also known as split testing, involves comparing two versions of an element (such as a webpage or email) to determine which one performs better. In an AB test, the audience is split into two groups: one group sees version A, and the other group sees version B. By analyzing the performance of each version, businesses can make data-driven decisions to improve conversions, engagement, and other key metrics.
Factors Influencing the Duration of an AB Test:
Sample Size
The sample size is one of the most important factors in determining the duration of an AB test. A larger sample size provides more reliable results and reduces the margin of error. However, achieving a large sample size can take time, especially for websites or campaigns with lower traffic.
Key Considerations:
Higher traffic sites can achieve a sufficient sample size more quickly.
Low traffic sites may need to run tests longer to gather enough data.
Use sample size calculators to estimate the number of participants needed.
Example: A high-traffic ecommerce site might reach a sufficient sample size in a few days, while a smaller blog might take weeks.
Expected Effect Size
The expected effect size refers to the magnitude of the difference you expect between the two versions. Smaller effect sizes require larger sample sizes to detect significant differences, which in turn can increase the duration of the test.
Key Considerations:
Large expected differences can be detected more quickly.
Small expected differences require more data and longer testing periods.
Set realistic expectations for the effect size based on previous tests or industry benchmarks.
Example: A major redesign of a landing page might show significant results quickly, whereas minor tweaks to button colors might take longer to show measurable differences.
Variability of Data
The variability or inconsistency in your data can impact how long it takes to reach reliable conclusions. High variability means that there are larger fluctuations in your data, which can necessitate a longer test duration to ensure accurate results.
Key Considerations:
High variability requires a longer test to account for fluctuations.
Stable, consistent data can shorten the required test duration.
Monitor variability during the test to adjust the duration if needed.
Example: A test on a highly seasonal product might need to run longer to account for daily or weekly sales fluctuations.
Statistical Significance
Statistical significance indicates whether the results of your test are likely due to chance or if there is a true difference between the two versions. Setting an appropriate significance level (commonly 0.05) ensures that you are confident in your results, but achieving this significance can take time.
Key Considerations:
Aim for a significance level of 0.05 to balance confidence and test duration.
Monitor the p-value throughout the test to gauge progress.
Be prepared to extend the test if statistical significance has not been reached.
Example: If the p-value remains high as the test progresses, you might need to extend the test duration to achieve statistical significance.
Business Cycle Considerations
The timing of your test in relation to your business cycle can affect how long it needs to run. Running tests during high-traffic periods, such as holidays or sales events, can help you reach a sufficient sample size more quickly.
Key Considerations:
Align tests with high-traffic periods for faster results.
Avoid running tests during abnormal business conditions that could skew data.
Consider the typical buying cycle of your audience.
Example: An ecommerce site might achieve faster results by running tests during the holiday shopping season, while a B2B company might need to account for longer decision-making cycles.
Conclusion:
The duration of an AB test is influenced by several factors, including sample size, expected effect size, data variability, statistical significance, and business cycle considerations. Understanding these factors can help you estimate the appropriate length of your test and ensure you gather reliable, actionable results.
By carefully planning your AB tests and monitoring progress, you can make data-driven decisions that optimize performance and drive better outcomes for your business.
© 2024 All Rights Reserved. AB Final.