Jun 25, 2024
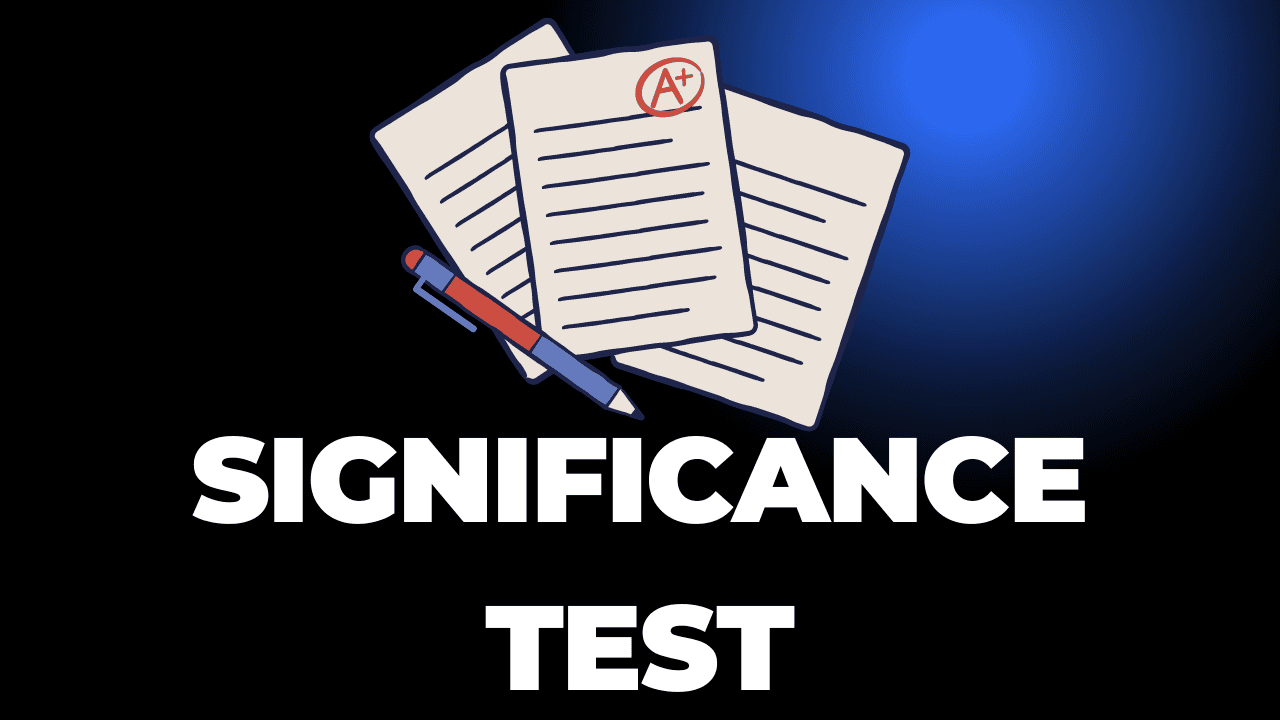
A/B testing is a method used to compare two versions of a webpage, app feature, or marketing campaign to determine which one performs better. A critical component of A/B testing is the significance test, which helps you determine whether the observed differences in performance between the two variants are statistically significant or simply due to random chance. Understanding and correctly applying significance tests are essential for making informed decisions based on your A/B test results.
The Role of Significance Testing in A/B Testing
1. Determining Statistical Significance
The significance test evaluates whether the differences in metrics (such as conversion rates, click-through rates, or engagement levels) between the control group and the treatment group are likely to be genuine effects rather than random fluctuations. This is typically expressed using a p-value, which measures the probability that the observed results could have occurred by chance.
2. Setting a Significance Level
The significance level, often denoted as alpha (α), is the threshold used to decide whether a result is statistically significant. Commonly, a significance level of 0.05 is used, meaning there is a 5% chance that the observed effect is due to random variation. If the p-value is less than the significance level, the result is considered statistically significant.
Key Concepts in Significance Testing
1. Hypothesis Formulation
Null Hypothesis (H0): Assumes there is no difference between the control and treatment groups. Any observed difference is due to chance.
Alternative Hypothesis (H1): Suggests that there is a significant difference between the control and treatment groups.
2. P-Value Interpretation
The p-value is the probability of observing a result at least as extreme as the one obtained, assuming the null hypothesis is true. A low p-value (typically ≤ 0.05) indicates strong evidence against the null hypothesis, leading to its rejection. Conversely, a high p-value suggests that the observed difference could plausibly be due to chance.
3. Confidence Intervals
Confidence intervals provide a range within which the true effect size is likely to fall, with a certain level of confidence (usually 95%). For example, a 95% confidence interval for a conversion rate difference might be 0.5% to 1.5%, indicating that the true difference is likely within this range.
Conducting a Significance Test
1. Selecting the Right Test
The choice of significance test depends on the data type and sample size. Common tests include:
t-Test: Used for comparing the means of two groups, assuming normal distribution and equal variances.
Chi-Square Test: Suitable for categorical data, testing the association between two categorical variables.
Mann-Whitney U Test: A non-parametric test for comparing two independent groups when the data is not normally distributed.
2. Performing the Test
Collect data from both the control and treatment groups. Use statistical software or online calculators to perform the significance test. Ensure the sample size is large enough to detect a meaningful difference, avoiding issues related to power and bias.
3. Interpreting the Results
Compare the p-value to the significance level (α). If p ≤ 0.05, reject the null hypothesis, concluding that the observed difference is statistically significant. If p > 0.05, you fail to reject the null hypothesis, indicating that any observed difference could be due to random chance.

Practical Considerations
1. Sample Size Determination
Ensure your sample size is adequate to detect a significant difference. Use sample size calculators to estimate the number of participants needed, considering the expected effect size, significance level, and desired power (commonly 80% or 90%).
2. Handling Multiple Comparisons
When conducting multiple A/B tests simultaneously, the chance of encountering false positives increases. Apply corrections such as Bonferroni or Holm-Bonferroni to control the family-wise error rate.
3. Considering Practical Significance
Statistical significance does not always imply practical significance. Evaluate the real-world impact of the observed differences, considering factors like user experience, cost, and business goals.
[BONUS]Advanced Techniques in Significance Testing
1. Bayesian A/B Testing
Unlike traditional frequentist approaches, Bayesian methods provide a probability distribution of the effect size, offering a more nuanced understanding of the evidence. Bayesian A/B testing can incorporate prior knowledge and update beliefs based on new data.
2. Multi-Armed Bandit Algorithms
These adaptive testing methods dynamically allocate traffic to different variants based on ongoing results, optimizing for the best-performing variant without waiting for the test to conclude. This approach balances exploration and exploitation, enhancing efficiency and speed.
3. Machine Learning Models
Advanced machine learning techniques, such as logistic regression, decision trees, or neural networks, can model complex relationships between variables and improve the accuracy of significance tests. These models can handle large datasets and capture non-linear interactions effectively.
Conclusion
Significance testing is a fundamental aspect of A/B testing, providing the statistical foundation needed to validate the results and make informed decisions. By understanding the principles of hypothesis testing, selecting appropriate tests, and interpreting p-values and confidence intervals, you can ensure that your A/B tests are robust and actionable.
Remember to consider practical significance, sample size, and the potential impact of multiple comparisons. Embrace advanced techniques to enhance the accuracy and efficiency of your testing process. By leveraging these insights, you can maximize the effectiveness of your A/B testing and drive meaningful improvements in your digital strategies.
© 2024 All Rights Reserved. AB Final.